
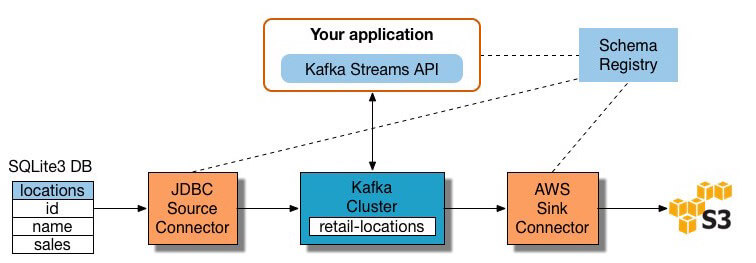
Format revision: Character set conversion, unit of measurement conversion, date/time conversion, etc.Deduplication: Identifying and removing duplicate records.Cleaning: Mapping NULL to 0 or "Male" to "M" and "Female" to "F," date format consistency, etc.Transformations prepare the data for analysis. Regardless of where in the process transformation takes place, it’s an important step in the analytic workflow. Today, this approach has enough advantages that it has become the default when projects decide to use the traditional model, this decision should come with real consideration of the costs and benefits.ĮTL in minutes > Extract from the sources that run your business. The ability for in-database transformations to be written in SQL, the data manipulation language of choice for most analysts.The increased performance and scalability of the modern analytics database.In recent years, there has been a shift toward transforming data within the warehouse rather than transforming it beforehand, primarily driven by two factors: Data is extracted and loaded into the analytics warehouse, and transformations are done there. In-warehouse data transformation – In this approach, the process flow changes to something more like ELT.Extracted data is moved to a staging area where transformations occur prior to loading the data into the warehouse. Multistage data transformation – This is the classic extract, transform, load process.Architecturally speaking, there are two ways to approach ETL transformation: Transformation refers to the cleansing and aggregation that may need to happen to data to prepare it for analysis.
